Data
You will be redirected to cenamweb.org in 15 second(s). The JINA-CEE website is not updated anymore. The archived website can still be reached through the CeNAM website.
Reconstructing Supernova Explosions with Physics-Based Machine-Learning Models
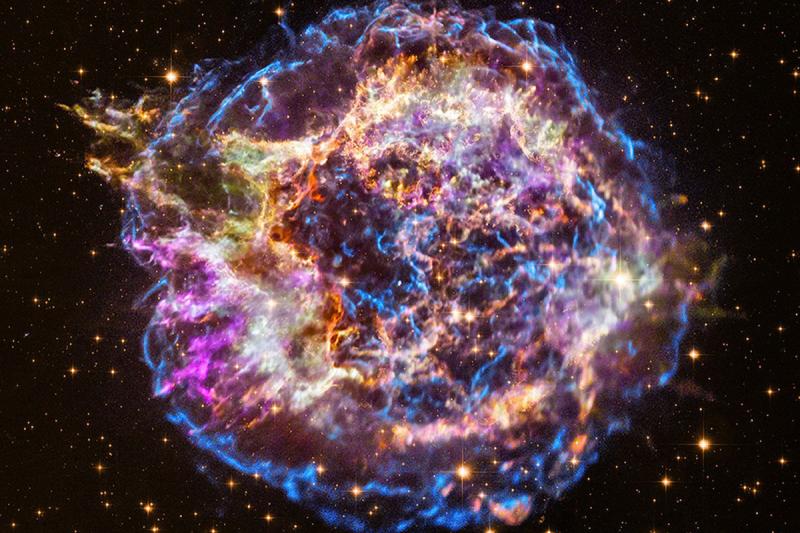
Contributed by Jack O'Brien and Wolfgang Kerzendorf (Michigan State University, USA)
Type Ia supernovae, the thermonuclear disruption of white-dwarfs in binary systems, have long been powerful tools for astronomers in understanding the evolution of the universe. Most famously they have served as powerful cosmic distance indicators leading to the Nobel-prize-winning discovery of Dark Energy. Despite their significance, the nature of their explosions remains a mystery. How and why a white-dwarf suddenly erupts into an explosion brighter than all of the stars in its host galaxy has been a matter of intense debate. Many progenitor scenarios have been proposed in order to explain the observed properties of Type Ia supernovae. We have developed a methodology and applied it to data that can discriminate between the progenitor scenarios.
Radiation-transport simulations are used to simulate observations of spectra which are then compared to real data. These models contain dozens of input parameters and detailed physics resulting in a highly complex parameter space. There may exist multiple solutions that produce similar looking synthetic spectra varying over orders of magnitude in parameters such as composition, temperature, and density. Getting a full picture of the space of all possible models that can describe an observation requires exploring millions of possible parameter combinations. Radiation-transport models take 10s of minutes to evaluate these parameters, making this problem computationally intractable, until now.
We have trained a machine-learning algorithm to run these radiative-transport simulations for us, substantially speeding up model evaluation. Our technique has reduced each parameter evaluation step from 10 minutes to fractions of a millisecond. Thus, the time required to perform the entire analysis has been reduced from 20 years to only 10 minutes! Exploring the relative likelihood of solutions in this space has revealed a complex and multi-modal parameter distribution. Some parameter combinations produce degenerate solutions and some elements can vary over orders of magnitude in relative abundance with little change on the resulting spectrum. Comparing our reconstruction of the Type Ia supernova SN2002bo to hydrodynamic simulations has revealed that the explosion best matches with a supersonic nuclear burning front, effectively ruling out the older turbulent models. Further constraining the possible scenarios for Type Ia supernovae will have profound implications on our understanding of their role in the evolution of the universe.
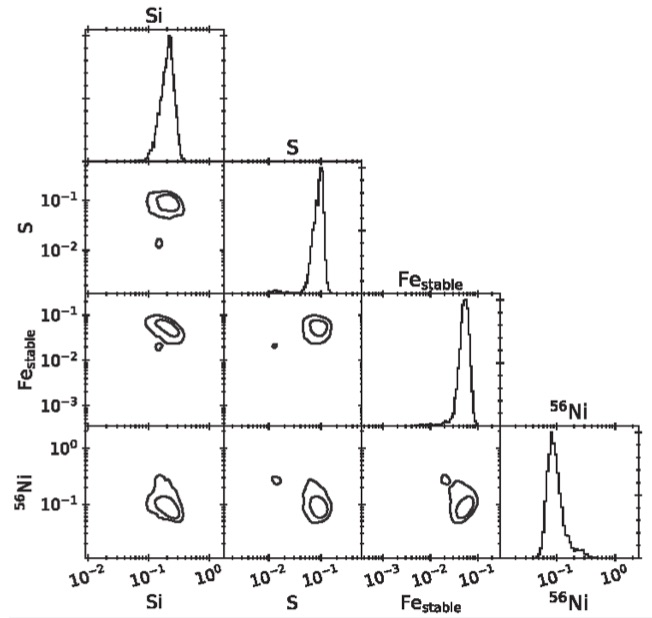